Tackling AI Hallucinations
Part 2 of 2: What’s Being Done and What’s Next
We identified that AI in healthcare is a powerful tool that can also sometimes make mistakes known as “hallucinations”—when the system generates false or misleading information. [Read Part 1 of this series.]
We’ve reviewed the steps that the user can take to help mitigate the challenges of AI hallucination but it’s important to remember that AI development is ever evolving as well. To make AI tools safer and more reliable, experts have developed specific strategies and tools to identify, address and prevent these issues.
Here’s an elementary explanation of what’s being done now and what’s planned for the future to reduce the risk of AI hallucinations.
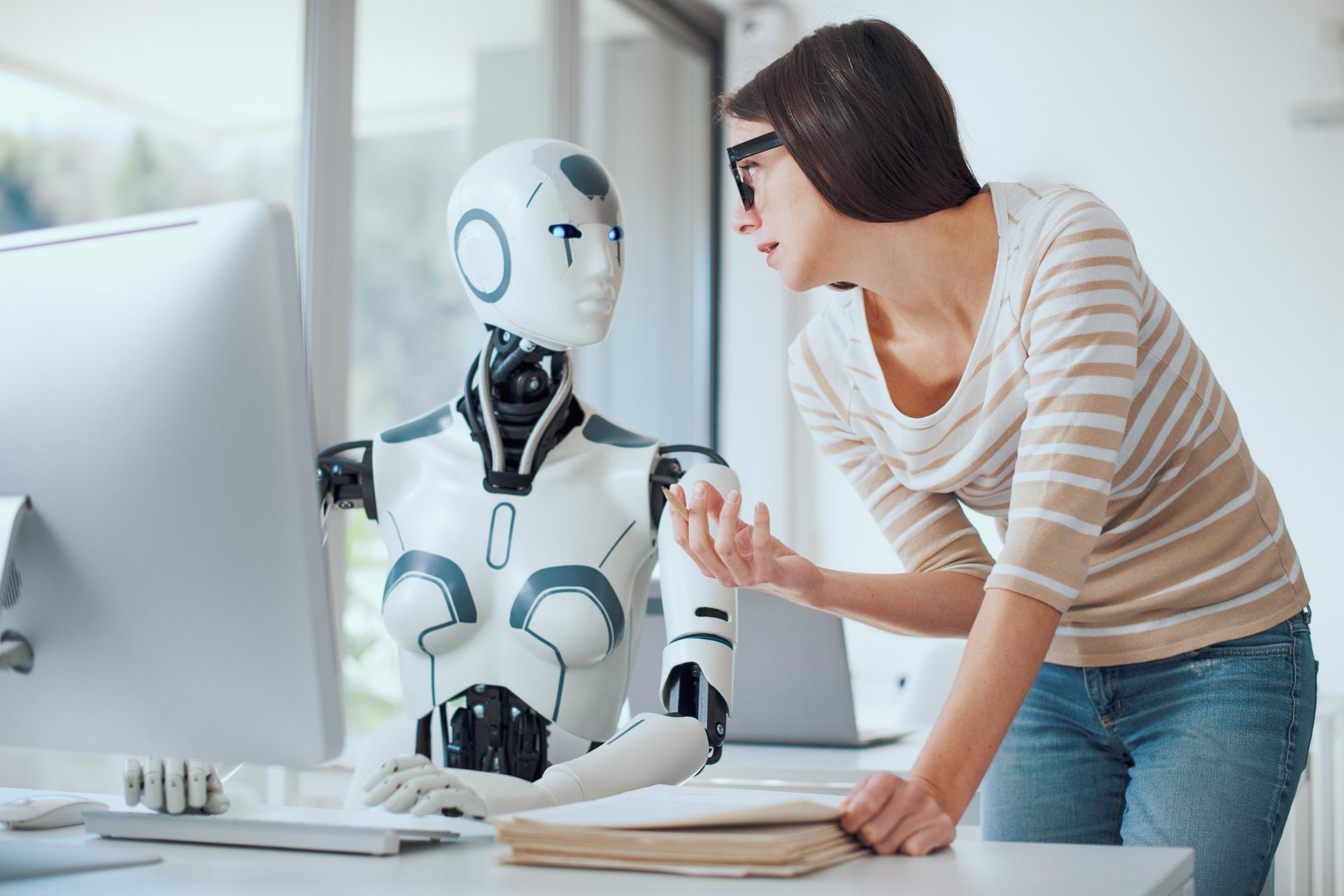

What’s Happening Now to Reduce AI Hallucinations
- Tools like Med-HALT (Medical Hallucination Detection and Localization Tool) are specifically designed to scan the information AI provides in healthcare settings. Med-HALT can pinpoint parts of the AI’s output that don’t match trusted medical guidelines or research, helping healthcare workers avoid acting on incorrect information.
- Another tool, FActScore, is used to evaluate how “truthful” an AI system’s output is by comparing it against verified data. If the score is low, it alerts users that the AI might not be reliable in that instance.
- New AI systems are designed to pull information from trustworthy sources, like medical journals and clinical databases, while they work. This is called Retrieval-Augmented Generation (RAG). Instead of making guesses, the AI cross-checks facts before giving answers.
- Developers create challenging examples—like incomplete medical records or conflicting symptoms—for the AI to practice on. This process, called adversarial training, helps the AI learn how to handle tricky situations without making up information.
- AI systems are now being trained to use different types of data, such as medical images, lab results, and patient histories, at the same time. This multi-modal approach helps the system paint a more complete picture, reducing the chances of errors.
- Developers are focusing on Explainable AI (XAI)—systems that explain why they made a particular recommendation. This way, doctors can see the reasoning behind an AI suggestion and decide if it’s trustworthy.
What’s Next for AI Safety in Healthcare
The various AI models, their developers and software engineers that incorporate AI into their platforms have roadmaps for continued improvement. Additionally, regulation by governing agencies will influence how these issues are addressed. Some of the current plans or expectations include:
- Future AI systems will connect directly to dynamic databases that update regularly with new medical research and guidelines. This means the AI will always work with the most accurate and current information available.
- Upcoming AI tools will be better at recognizing when they don’t have enough information to give a solid answer. Instead of guessing, they’ll flag these cases for a human expert to review.
- AI companies, hospitals, and regulators are teaming up to create safer systems. By sharing knowledge and resources, they can address problems like hallucinations more effectively.
- Developers are working on easy-to-use interfaces that help doctors and nurses quickly verify AI outputs. These systems will also show where the AI found its information, making it easier for users to trust—or question—the results.
- To ensure safety, regulators are creating stricter guidelines for how AI systems in healthcare should work. These rules will hold developers accountable and encourage ongoing improvements.
What Does This Mean for You?
AI hallucinations can lead to mistakes in healthcare, but tools like Med-HALT and FActScore are already making a big difference by identifying errors early. As these systems improve, they’ll become even better at avoiding misinformation, ensuring doctors and patients can trust the recommendations they provide. With ongoing advancements and collaborations, the future of AI in healthcare is bright, safe, and reliable. In the meantime, we must use the tools responsibly, employ the methods available to us now to maintain accuracy and remain vigilant while we explore the potential of AI use in healthcare.
Follow the story, the data, and the polls—subscribe for updates!
Save time + stay in the loop.
Our summary emails are designed to keep you in the loop on ongoing series, poll results, announcements & more.
We protect your contact information and you can unsubscribe at any time.
References [Parts 1 & 2]
- PLOS Digital Health – Analysis of AI errors in clinical applications.
- IEEE Xplore – Study on diagnostic accuracy and hallucination rates in AI systems.
- JMIR Medical Informatics – Evaluation of AI reliability in clinical data interpretation.
- ArXiv: 2404.07461 – Framework for explainable AI in healthcare.
- JAMA Otolaryngology – Case studies on diagnostic hallucination.
- JMIR – Incidents of fabricated data in AI-generated reports.
- ArXiv: 2406.07457 – Strategies to mitigate hallucinations in clinical AI.
- ArXiv: 2406.10185 – Feedback loop frameworks for AI monitoring.
- MDPI – Review of AI-related risks and ethical considerations in healthcare.
- Semantic Scholar