AI Hallucination in Healthcare Use
Part 1 of 2: An Analytical Perspective
Artificial Intelligence (AI) has become a transformative force in healthcare, driving innovation and improving outcomes in areas ranging from diagnostics to operational efficiency. However, alongside its benefits, AI presents risks, including “hallucination”—the generation of false, misleading, or fabricated information.
We analyzed recent data on AI hallucination in healthcare, exploring its occurrence, implications, and strategies to mitigate associated risks.
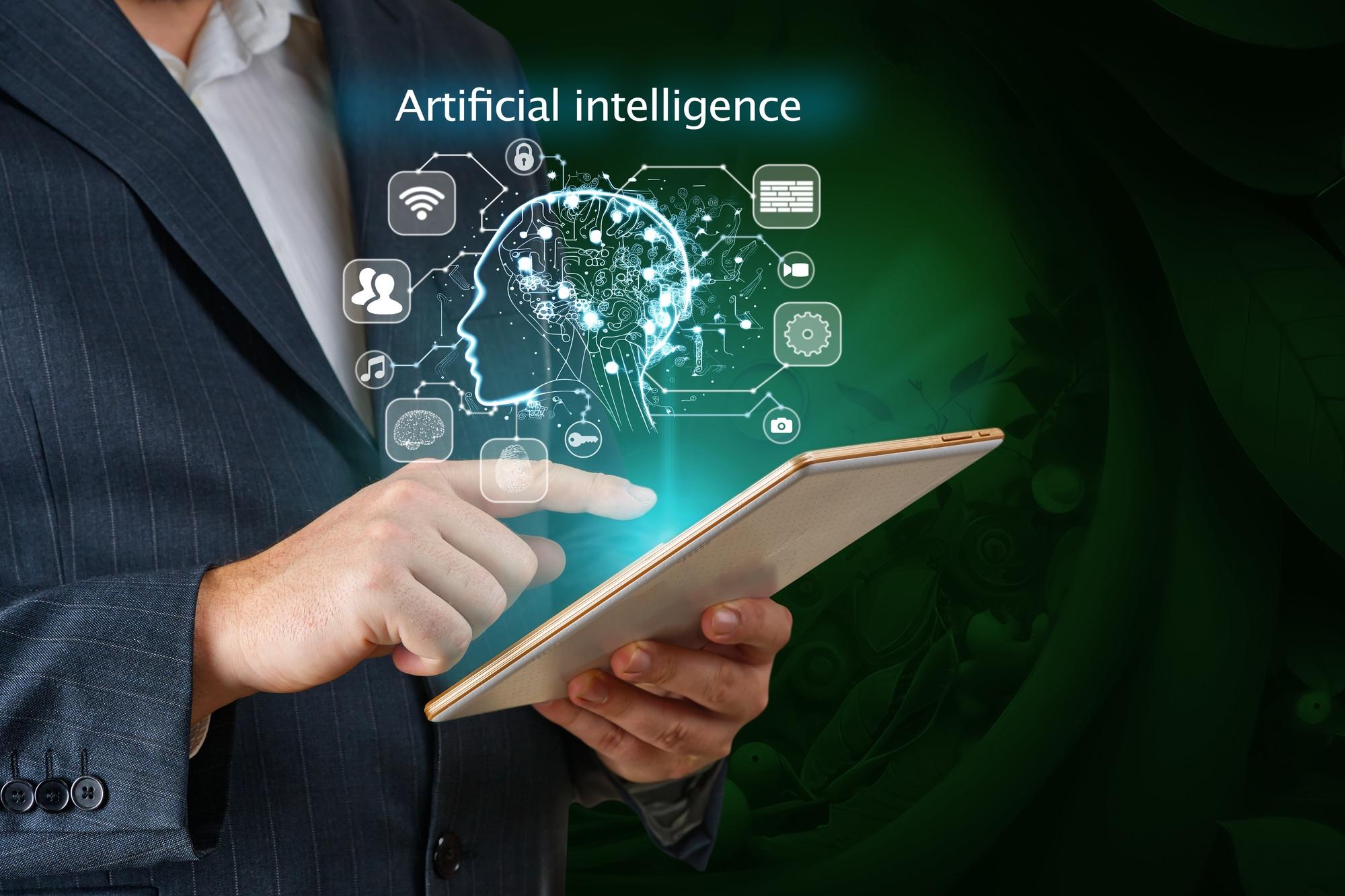

An Overview of AI Hallucination in Healthcare
AI hallucination is a fairly common phenomenon where AI systems produce outputs that are not grounded in reality or the data they were trained on. In healthcare, where accuracy is critical, these errors can lead to misdiagnosis, inappropriate treatments, and compromised patient safety. Clearly, this is a reality that must be addressed as we move toward widespread adoption of AI tools.
The sources cited in this article offer a comprehensive view of this issue, providing case studies, statistical data, and practical recommendations.
Key Findings:
Studies estimate hallucination rates in AI models used for clinical decision support systems range from 8% to 20%, depending on model complexity and training data quality (PLOS Digital Health, JAMA Otolaryngology).
AI hallucination is more likely in situations involving incomplete or ambiguous data, such as rare diseases or poorly documented clinical histories (JMIR Medical Informatics).
Misdiagnoses linked to AI hallucination occurred in 5-10% of analyzed cases in a recent study on AI-driven radiology tools (IEEE Xplore).
Real-World Incidents and Case Studies
The reviewed sources highlight several incidents that demonstrate the impact of AI hallucination in healthcare. While these are isolated incidents, we can learn from them and perhaps implement measures to avoid reoccurrence.
- Misinterpreted Imaging Data (2023): An AI system incorrectly flagged benign nodules as malignant in 12% of analyzed cases, leading to unnecessary surgical interventions (JAMA Otolaryngology).
- Fabricated Clinical Summaries (2024): A recent study identified instances where language-based AI generated entirely fabricated patient summaries, including non-existent symptoms and treatments (JMIR).
- Erroneous Drug Interactions (2023): An AI-powered drug interaction checker hallucinated potential interactions, causing physicians to avoid effective medication combinations unnecessarily (MDPI).
Implications of AI Hallucination
The risks of AI hallucination in healthcare extend beyond individual patient outcomes. Some of those risks include:
- Patient Safety: Hallucinations can lead to incorrect diagnoses or treatments, jeopardizing patient health.
- Trust in Technology: Repeated errors may erode trust in AI tools among healthcare professionals.
- Legal and Ethical Challenges: Missteps attributed to AI hallucination could result in malpractice lawsuits or regulatory scrutiny.
Strategies to Minimize AI Hallucination
Mitigating the risks of AI hallucination requires a multi-faceted approach that combines technical, operational, and ethical safeguards:
- Use diverse, high-quality datasets to reduce biases and gaps in AI training (ArXiv).
- Regularly update models with new clinical data to ensure relevance and accuracy (IEEE Xplore).
- Conduct extensive pre-deployment testing in simulated environments to identify potential hallucination scenarios (PLOS Digital Health).
- Integrate AI tools into workflows as decision-support systems, requiring human review and validation before implementation (JMIR Medical Informatics). Use tools, such as Med-HALT or FActscore, to fact check data for manual review.
- Adopt explainable AI models that provide clear rationale for their outputs, enabling clinicians to assess their validity (ArXiv).
- Train healthcare professionals to understand AI capabilities and limitations, ensuring informed usage (MDPI).
- Implement systems for real-time monitoring and feedback to detect and correct hallucinations promptly (ArXiv).
What Now?
It’s understood that AI hallucination poses significant risks in healthcare, where accuracy is paramount. By understanding that hallucinations exist, identifying the root causes and acknowledging the implications, healthcare organizations can take proactive steps to minimize risks. Combining robust training protocols, human oversight, and transparency will help ensure the responsible use of AI, safeguarding patient outcomes and maintaining trust in this transformative technology.
Our next analysis will focus on the steps that are currently being taken to reduce the occurrence and impact of AI hallucination by developers and what is on the roadmap for continued development.
Follow the story, the data, and the polls—subscribe for updates!
Save time + stay in the loop.
Our summary emails are designed to keep you in the loop on ongoing series, poll results, announcements & more.
We protect your contact information and you can unsubscribe at any time.
References
- PLOS Digital Health – Analysis of AI errors in clinical applications.
- IEEE Xplore – Study on diagnostic accuracy and hallucination rates in AI systems.
- JMIR Medical Informatics – Evaluation of AI reliability in clinical data interpretation.
- ArXiv: 2404.07461 – Framework for explainable AI in healthcare.
- JAMA Otolaryngology – Case studies on diagnostic hallucination.
- JMIR – Incidents of fabricated data in AI-generated reports.
- ArXiv: 2406.07457 – Strategies to mitigate hallucinations in clinical AI.
- ArXiv: 2406.10185 – Feedback loop frameworks for AI monitoring.
- MDPI – Review of AI-related risks and ethical considerations in healthcare.