TRUST | EXPERTISE | INNOVATION | OUTCOMES
LEVERAGED EXPERTISE
Payers, Providers & Government Agencies turn to us for results they can count on.
OVER 20 YEARS EXPERIENCE
Physician Authorization Reviews
Peer to Peer Appeals
Utilization Management Reviews
Nurse Augmentation
NCQA and URAC Accreditation Assistance Consulting
Office of Inspector General Reviews
Appeals Review
Performance Improvement Services
Specialist Review
Utilization Management and Peer Review Software
Medical Director Services
Business Intelligence Services
Utilization Management and Peer Review Software
Why BHM?
For over 20 years, our unwavering focus on serving clients with excellence has set us apart.
Here are a few ways we demonstrate that commitment.
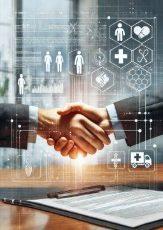
Trust
Reliable Partner
We’ve built our reputation on integrity, transparency, and delivering dependable services that organizations trust to support critical decisions in cost effective care.
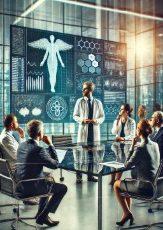
Expertise
Industry Knowledge
Our team of seasoned professionals and clinical experts provide unparalleled insights and guidance tailored to meet the complex needs of healthcare payers and providers.
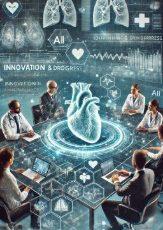
Innovation
Driving Progress
We combine advanced technologies and forward-thinking strategies to deliver efficient, scalable solutions that enhance outcomes and streamline processes.
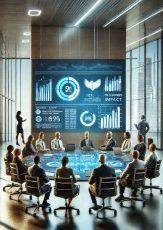
Outcomes
Measurable Impact
Our systems are designed to employ data-driven insights to help organizations improve quality, reduce costs, and meet compliance standards for lasting success.